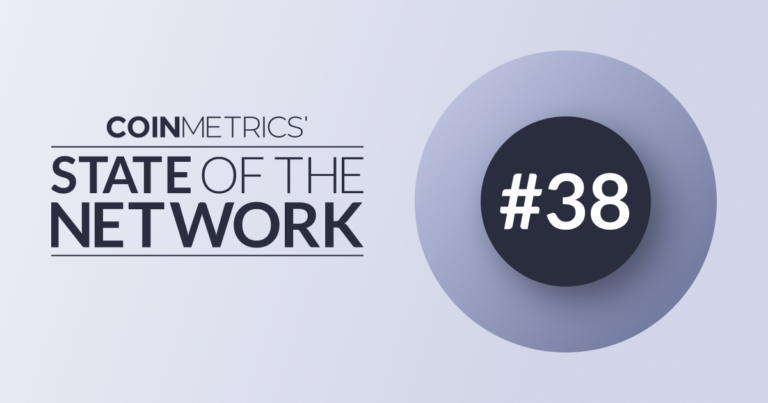
Analyzing Crypto Supply Distribution Patterns
Those who control the wealth often control the power. But up until now, wealth distribution has been relatively hard to
Those who control the wealth often control the power. But up until now, wealth distribution has been relatively hard to
Cryptoassets represent a significant innovation in the evolution of money and the modern financial system. Valuing cryptoassets remains very much
[fusion_builder_container type=”flex” hundred_percent=”no” equal_height_columns=”no” hide_on_mobile=”small-visibility,medium-visibility,large-visibility” background_position=”center center” background_repeat=”no-repeat” fade=”no” background_parallax=”none” parallax_speed=”0.3″ video_aspect_ratio=”16:9″ video_loop=”yes” video_mute=”yes” border_style=”solid”][fusion_builder_row][fusion_builder_column type=”1_1″ type=”1_1″ background_position=”left top” border_style=”solid”
In traditional capital markets, corporate actions are events that could bring a change to the securities (equity or debt) of